Nicholas Baker
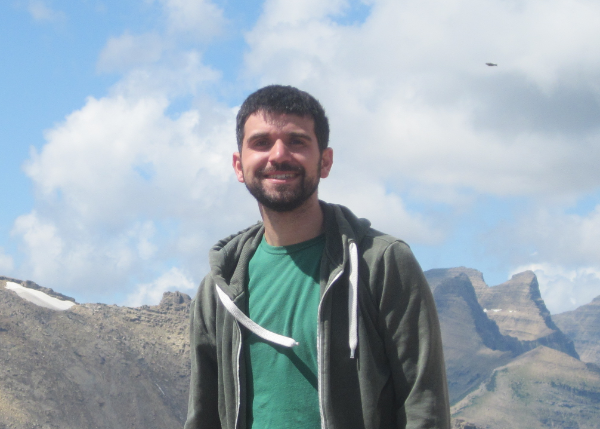
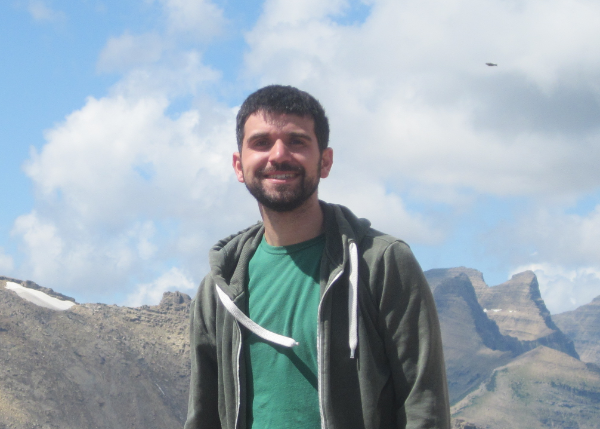
Title: Assistant Professor
Office: Coffey Hall 222
Phone: 773.508.3012
Email: nbaker1@luc.edu
Background Information
BA: Johns Hopkins University
PhD: University of California, Los Angeles
Post-doc: York University
Additional Information
Human Perception Lab
Classes Taught
PSYC 240 Psychology and Biology of Perception
PSYC 316 Lab in Experimental Psychology: Sensation and Perception
Research Interests
In my research, I study how the mind perceives and recognizes shapes and objects from visual inputs. Inspired by classic work by the Gestalt psychologists, one of the central themes of my research is that abstract relations are more important in object perception than encoding the physical properties of an object. For example, you can look at a photograph of a dog, a silhouette or a dog, or even a few well chosen dots from the outline of a dog, and they will all look like the same dog as long as the spatial relations between elements are preserved. These abstractions are evident everywhere, from when we compress the complex contour of a pine into a triangular shape to when a few well-chosen brushstrokes from Picasso look like a penguin. I research the information processing systems that transform a physical contour into an abstract shape representation.
My research can be subdivided into three broader categories. First, I study the nature of shape abstraction in human vision. Second, I compare human vision to state-of-the-art computer vision algorithms to see how they differ in their ability to extract abstract relations of objects. Finally, I model the computational systems in the mind that transforms a physical object boundary into an abstract shape representation.
Recent Publications
Baker, N., garrigan, P., & Kellman, P.J. (2020). Constant curvature segments as building blocks of 2D shape representation. Journal of Experimental Psychology: General.
Baker, N., Lu, H., Erlikhman, G., & Kellman, P.J. (2020). Local features and global shape information in object classification by deep convolutional neural networks. Vision research, 172, 46-61.
Baker, N., Lu, H., Erlikhman, G., & Pellman, P.J. (2018). Deep convolutional networks do not classify based on global shape. PLoS computational biology, 14(12), e1006613.
Baker, N., & Kellman, P.J. (2018). Abstract shape representation in human visual perception. Journal of Experimental Psychology: General, 147(9), 1295.